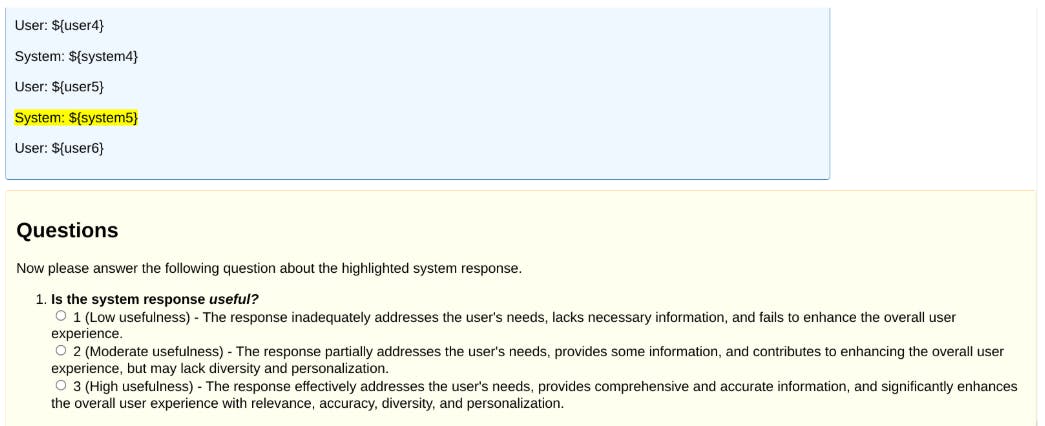
How to Improve Crowdsourced Labels for Dialogue Systems
9 Apr 2025
Explore supplementary materials supporting the main paper—Context Does Matter: Implications for Crowdsourced Evaluation Labels in Task-Oriented Dialogue Systems
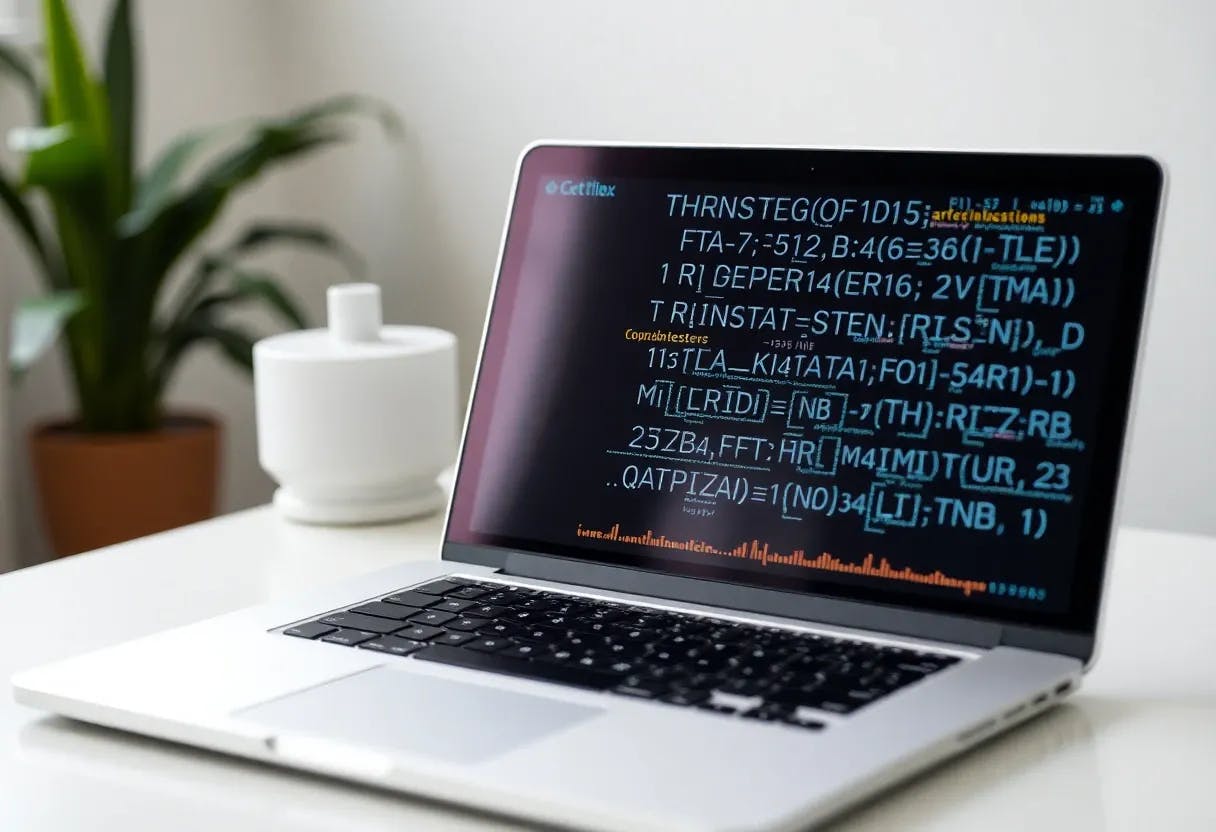
How to Improve the Accuracy of Online Ratings for AI Chatbots and Virtual Assistants
9 Apr 2025
This study shows that minimal context and heuristic methods can boost crowdsourced label consistency for relevance and usefulness in TDS evaluations.
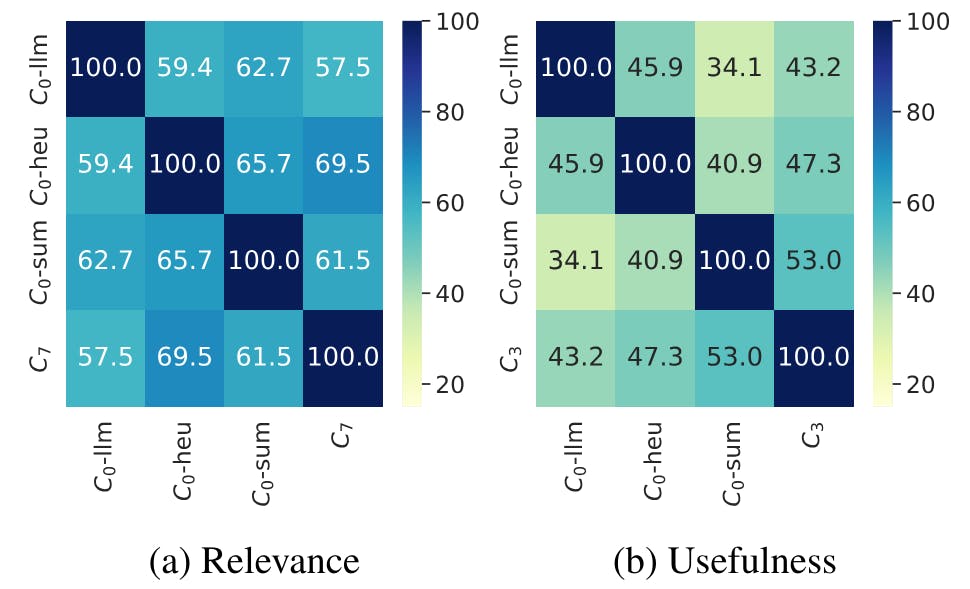
When Rating AI Chatbots, More Context Isn't Always Better
8 Apr 2025
Expanding context improves label consistency in TDS evaluations, but too much context can confuse annotators.
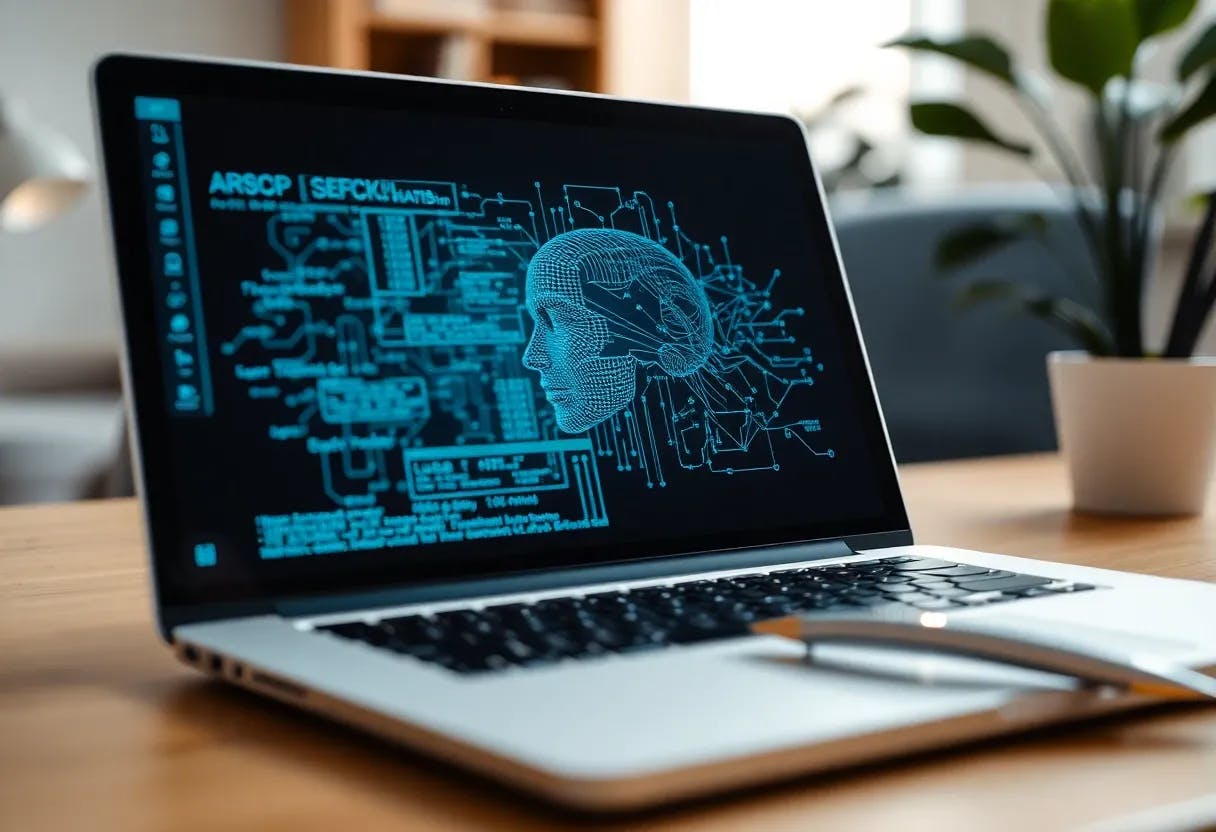
Can AI-Generated Context Improve the Quality of Crowdsourced Feedback?
8 Apr 2025
Heuristic-generated context boosts crowdsourced label quality and consistency, outperforming LLM-based methods for both relevance and usefulness evaluations.
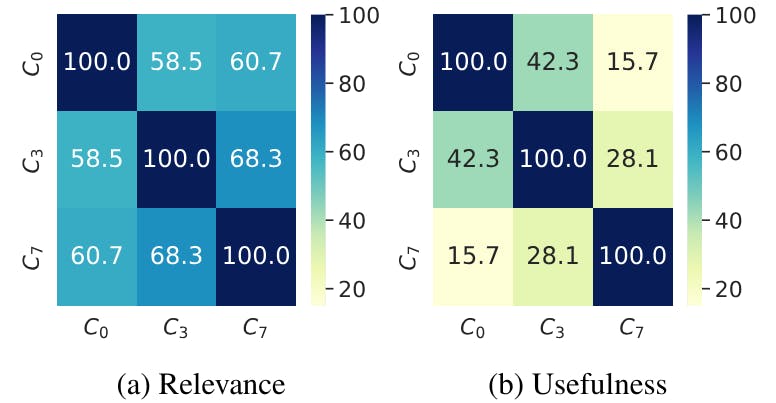
The Surprising Effects of Minimal Dialogue Context on AI Judgment
8 Apr 2025
Increasing dialogue context boosts agreement on relevance ratings, but can cause inconsistency in usefulness judgments due to complex user feedback.
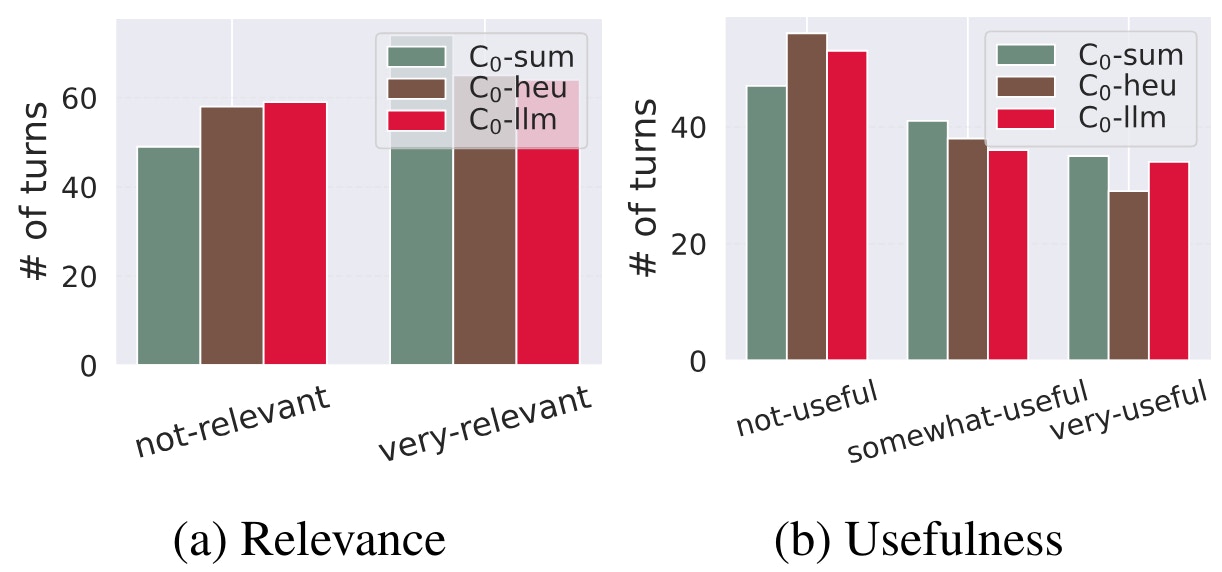
Study Finds AI Responses Rated Higher When Context is Limited
7 Apr 2025
Missing context skews AI ratings; summaries improve relevance accuracy, but usefulness still suffers due to limited understanding of user intent.
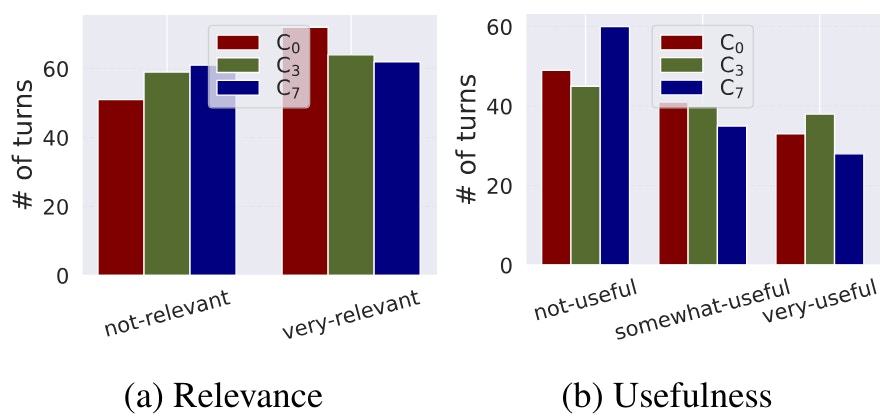
How Context Changes the Way We Rate AI Responses
7 Apr 2025
Study examines how dialogue context affects crowdsourced AI evaluation, testing different contexts using LLMs and heuristics for improved consistency.
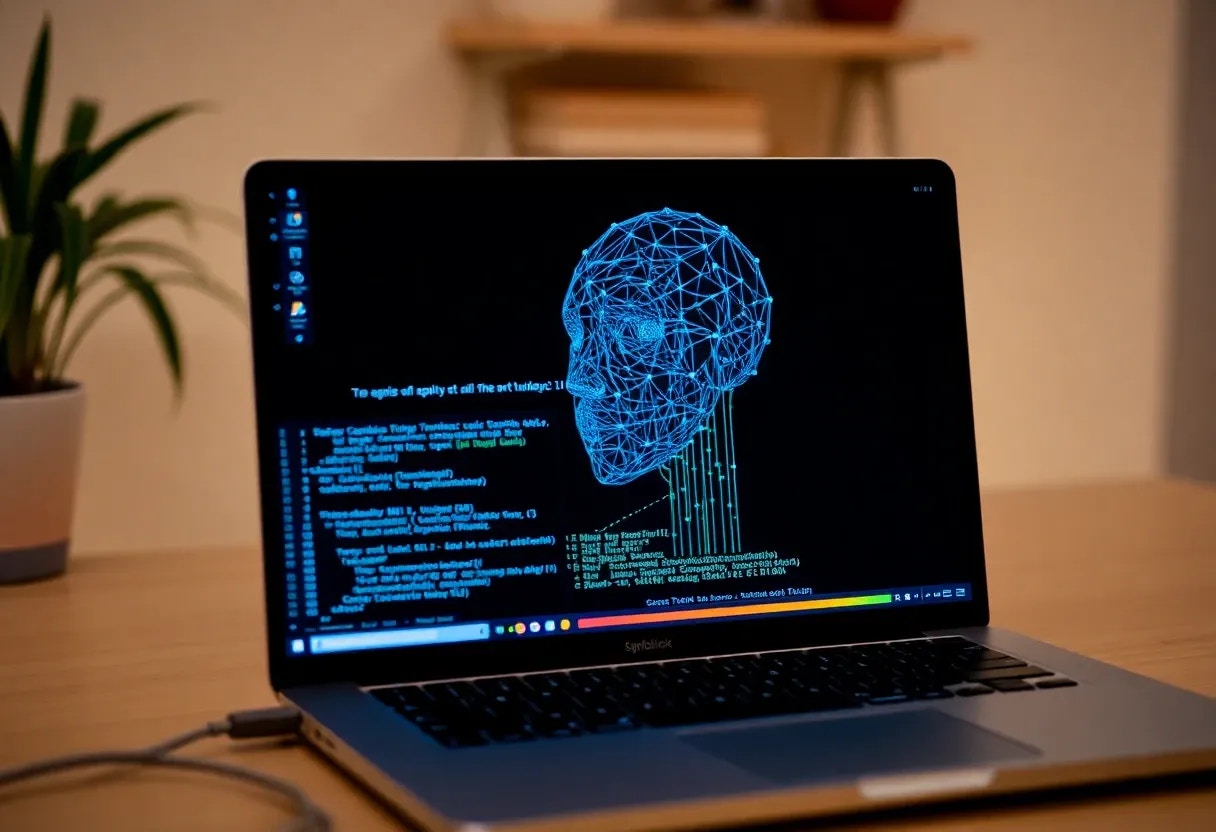
Can LLMs Improve Crowdsourced Evaluation in Dialogue Systems?
7 Apr 2025
Study explores how varying dialogue context impacts crowdsourced judgments and how LLMs can improve relevance and usefulness ratings in chatbot evaluations.
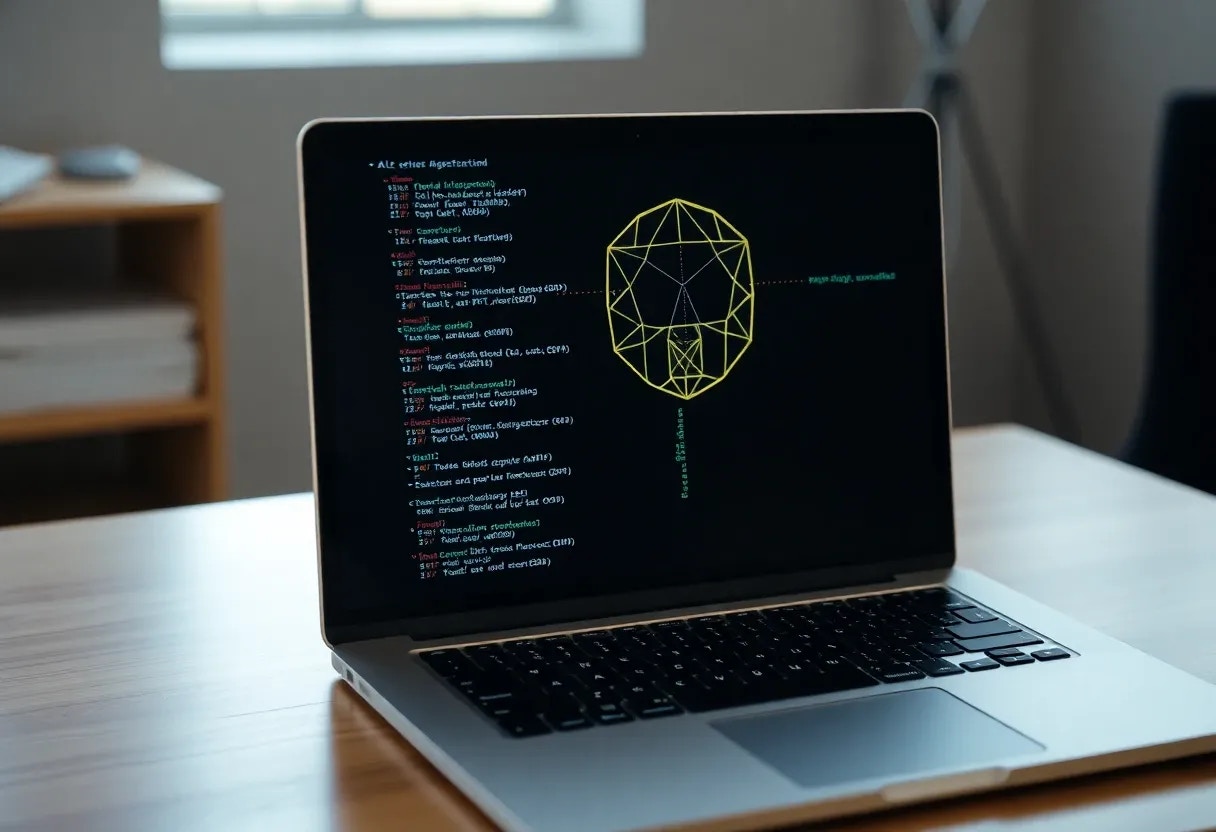
When Labeling AI Chatbots, Context Is a Double-Edged Sword
7 Apr 2025
Context impacts label quality in chatbot evaluations. LLM summaries boost annotator accuracy while reducing effort and bias.